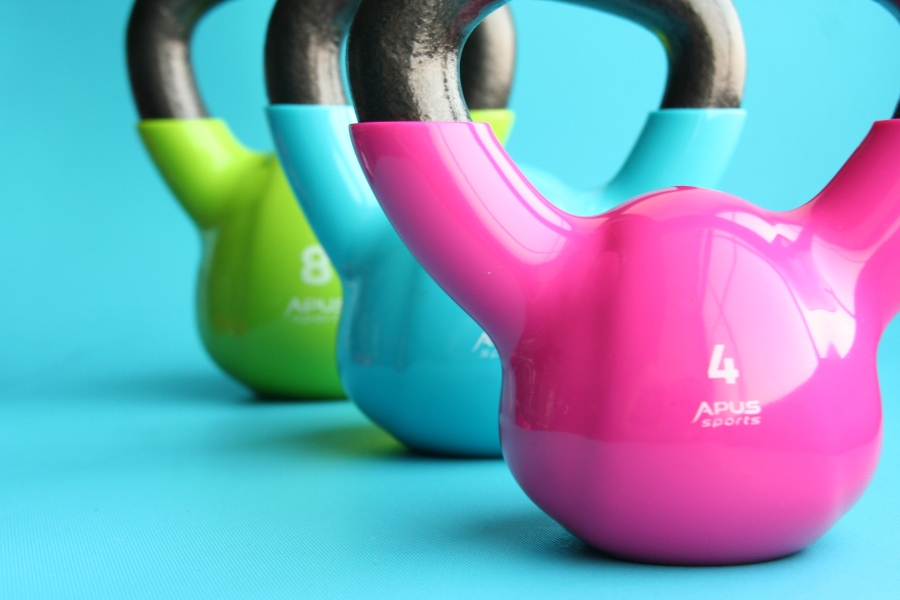
If you’ve ever shopped online on Amazon, you’ll notice product recommendations everywhere on their site and in your inbox. And, if you’re paying attention, these recommendations are usually highly relevant.
This is not a coincidence.
Amazon uses machine learning processes to run its recommendation engine to recommend highly relevant products to targeted users based on:
- What’s currently in their shopping cart
- Shopping history
- What other users buy when purchasing similar products
- Customer ratings and reviews
And by using these data inputs, they’re recommendation algorithms have proved highly effective, resulting in about 35% of all online purchasing coming via its recommendation engine.
Companies like Netflix have also used machine learning algorithms to recommend content, which has helped them save roughly $1 billion dollars a year in service cancellations.
What links these companies--and the fitness management industry--are the large amounts of 1st party data at their disposal.
With machine learning at the forefront of innovation in club management, it’s only natural that it be applied to increasing point-of-sale revenue.
Here we’ll go over how product recommendations work and how they can help clubs increase revenue by providing their staff with the data to offer the right product to the right customer at the point-of-sale.
Club Product Recommendations
It’s no secret that selling merchandise at your club can be a significant revenue booster. People are often times in need of immediate refreshment after their workouts, are too busy to stop by a local retailer, or simply like to wear your club’s apparel.
Whatever the reason, some clubs’ merchandise revenue streams are so significant they’re staples in their business plans.
And why wouldn’t they be as they can account for a large portion of a clubs revenue outside of contracts.
Whether you’re struggling with earning profitability with inventory sales at the front desk or thinking about getting started, product recommendations based on machine learning can bring in additional revenue just by knowing which products to offer which clients.
The goal really isn’t to create buyers from non-buyers, but to increase the average order size from the existing buyers.
The Need
If you’re like one of the people described above who purchases products within your club, then you know the value of a personalized approach.
Front desk staffers can often times offer your products that you frequently buy because they either remember or you have those notes retained in the system, like it is in the PG PoS below.
All of this stems just from associating products purchased with an account and the frequency of attendance vs. the amount of times a product is purchased.
This information allows your front desk personnel to always have a personalized approach when it comes to selling inventory items upon user check-in or check-out.
And your customers will appreciate it as it will save time for them as well as your staff.
Usually, if clients don’t want anything else, they won’t ask. However, we’ve all encountered situations where another product was offered at check-out we didn’t realize that we wanted or needed, until it was presented to us.
This is, of course, the crux of recommendation engines like Netflix’s and Amazon’s.
The trick in applying it to front-desk transactions is offering the right products to the right people.
This is where machine learning comes in to play.
Making Smart Recommendations
All good recommendation algorithms will first need data to analyze and process. That’s why we recommend using a system in which all club transactions are logged and monitored.
Having entire transaction histories across all clubs and clients gives the machine learning algorithms a good baseline in which to find patterns between customer segments and products purchased.
As one of the foundations for any machine learning process, pattern recognition will find correlations between different data inputs and signify a relationship between them.
However, if the data points are unrelated, you can end up with some wild correlations that highlight the old saying “correlation doesn’t mean causation”, an example being in the image below.
But if we’re looking at a defined outcome, such as a purchase completion among products sold in a particular fitness center, we can find patterns of what items were bought in tandem with other items and make our recommendations accordingly.
To do this, we might want to first segment our users, for example into male vs. female.
According to a recent study, men and women have vastly different reasons for completing transactions:
“For men, good services mean helping them find the right item and helping them get through checkout quickly. For women, customer satisfaction is strongly linked to a shop’s willingness to share expert advice (demonstrate familiarity with the product) and the shop’s ability to help them determine which products suit best.”
Thus, we will segment our users into genders to more effectively recommend products to them.
For example, we see that within a fitness club, both men and women frequently buy orange flavored energy drinks; however, men frequently purchase protein powder while women purchase protein bars when also purchasing the energy drink.
Therefore, these patterns would be recognized and after someone requests a product, the front desk staff will then offer the corresponding product that most frequently is purchased with the energy drink.
These recommendations will occur as rule-based recommendations, simply meaning that:
IF Female + BUYS Orange Energy Drink ---> RECOMMEND Protein Bar
The process may seem simple enough, but again it takes a lot of computing power that involves pattern recognition and historical data processing to inform the rules that ultimately display which products to recommend to which clients.
Conclusion
Increasing the average order size of your existing buyers should be a revenue growth strategy all clubs with inventories implement.
Now with the help of machine learning processes at the point-of-sale, front-desk personnel can offer clients products with data-backed insights to ultimately increase a clubs front-desk profits.